Tag Archives: Machine Learning
I am completely OK with this
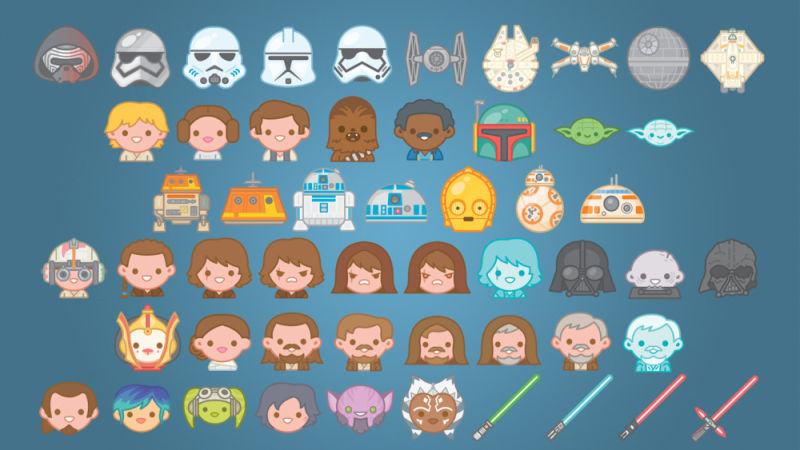
Now this I have no problem with whatsoever, although it hints at a larger issue. Researchers at University College London have discovered a dormant but massive Twitter botnet comprised of an estimated 350,000 fake accounts that does nothing but tweet out random quotes from Star Wars novels.
They discovered it quite by accident while taking a pure random sample of English-speaking Twitter accounts. It’s important to note the importance of this sampling method, as other methods of sampling might bias the results in favor of those accounts that are more active or have more followers. Their one percent sample resulted in approximately six million accounts.
Once their random sample was complete, they plotted the geographic distribution of these users, and they discovered something curious. Many of the tweets formed an almost perfect rectangle along latitude/longitude lines, including open, uninhabitable places like frozen tundra and bodies of water. They conjecture the shape was deliberate to mimic where English-language tweets are most likely to originate, and hide them within the clutter of legitimate Twitter users Tweet flood.
Upon further investigation, the researchers found another surprise. All these Twitter accounts did was tweet out random passages from Star Wars novels. They also never retweet, they send out very few tweets (around ten total) and list ‘Twitter for Windows Phone’ as the tweet source. As much as I hate to say it, that is also likely a ploy to get them to stay under the radar as much as possible because of that platform’s significantly low user base.
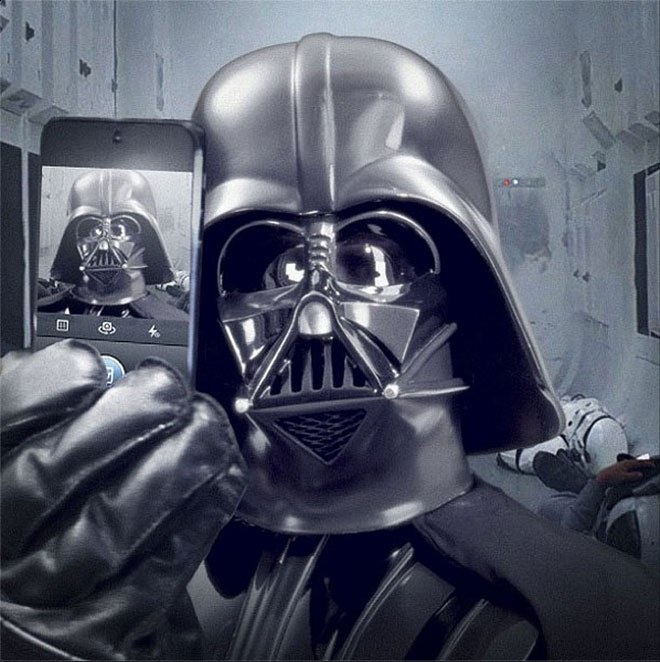
It’s not Twitter, but Darth Vader actually posted this on Instagram. Seriously. He doesn’t even care about that stormtrooper behind him.
Using a machine-learning word association approach (a ‘classifier,’ although classifiers are not limited to word association), it found that actual users had a very wide distribution of word choice, while the bots used words almost entirely related to Star Wars. Additionally, the platform percentages were evenly distributed for the most part among real users while the botnet was one hundred percent Twitter for Windows Phone. When the numbers are examined, the botnet is easy to see.
The authors then discuss the implications. Clearly, a dormant, low-activity Star Wars-themed Twitter botnet is not a big deal. However, if the creator decided to reactivate the botnet in order to create a spam network, send malicious messages, or use it for other nefarious purposes, they could. I personally don’t believe that will happen as it likely would have already, however as the authors also note, the botnet went out of its way to stay under the radar.
One of the things I find most interesting about it all is that the authors hint they found another, even more massive Twitter botnet using the same approach, which they will be reporting on at a later date.
Really interesting stuff, and touches on the impact of social media, machine learning and AI, cybersecurity, and geolocation/geotagging just to start (as well as the curious motivations of this particular botnet’s creator). I very much recommend giving it a read.